the Creative Commons Attribution 4.0 License.
the Creative Commons Attribution 4.0 License.
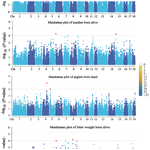
Genome-wide single nucleotide polymorphism (SNP) data reveal potential candidate genes for litter traits in a Yorkshire pig population
Yu Zhang
Jinhua Lai
Xiaoyi Wang
Mingli Li
Yanlin Zhang
Chunlv Ji
Qiang Chen
Shaoxiong Lu
The litter trait is one of the most important economic traits, and increasing litter size is of great economic value in the pig industry. However, the molecular mechanisms underlying pig litter traits remain elusive. To identify molecular markers and candidate genes for pig litter traits, a genome-wide association study (GWAS) and selection signature analysis were conducted in a Yorkshire pig population. A total of 518 producing sows were genotyped with Illumina Porcine SNP 50 BeadChip, and 1969 farrowing records for the total number born (TNB), the number born alive (NBA), piglets born dead (PBD), and litter weight born alive (LWB) were collected. Then, a GWAS was performed for the four litter traits using a repeatability model. Based on the estimated breeding values (EBVs) of TNB, 15 high- and 15 low-prolificacy individuals were selected from the 518 sows to implement selection signature analysis. Subsequently, the selection signatures affecting the litter traits of sows were detected by using two methods including the fixation index (FST) and θπ. Combining the results of the GWAS and selection signature analysis, 20 promising candidate genes (NKAIN2, IGF1R, KISS1R, TYRO3, SPINT1, ADGRF5, APC2, PTBP1, CLCN3, CBR4, HPF1, FAM174A, SCP2, CLIC1, ZFYVE9, SPATA33, KIF5C, EPC2, GABRA2, and GABRA4) were identified. These findings provide novel insights into the genetic basis of pig litter traits and will be helpful for improving the reproductive performances of sows in pig breeding.
- Article
(4617 KB) - Full-text XML
-
Supplement
(1214 KB) - BibTeX
- EndNote
Litter traits, mainly including the total number born (TNB), the number born alive (NBA), piglets born dead (PBD), and litter weight born alive (LWB) (Wu et al., 2018), are some of the most important economic traits in the pig industry. Improving litter size is a primary objective and has been a central focus in breeding programs for several decades, particularly in well-organized breeding systems like the Canadian Centre for Swine Improvement (http://www.ccsi.ca/, last access: 20 October 2022) (Zhang et al., 2019). However, due to the low heritability of these traits and the cumulative small effects of multiple genes, genetic improvement for these reproductive traits is extremely limited by traditional breeding techniques based on the best linear unbiased predictions (BLUPs) (Holm et al., 2004). Therefore, it is necessary to further unravel the underlying genetic architecture of these traits to improve the breeding schemes. With the development of high-throughput genotyping technology and the improvement in genetic statistical methods, some methods have been widely used in the genetic dissection of important economic traits in livestock, such as a genome-wide association study (GWAS) and selective sweep analysis.
Since the publication of domestic pig genome data in 2005 (Wernersson et al., 2005), GWASs have been widely used to identify molecular markers, genes, and quantitative trait loci (QTL) for reproductive traits in pigs, such as litter size (Uzzaman et al., 2018; Jiang et al., 2020; Chen et al., 2019), the number of teats (Bovo et al., 2021; Moscatelli et al., 2020), ovulation rate (Schneider et al., 2014; He et al., 2017), and gestation length (Hidalgo et al., 2016; See et al., 2019). In addition, phenotypic variability offers the opportunity to investigate the molecular basis of differentiation between populations (D'Alessandro et al., 2020). In the past decade, selection signature has been used to identify candidate regions of chromosomes of phenotypic traits in pigs. For instance, Li et al. (2020) identified 1017 selective windows containing 280 genes by comparing genomes of Large White pigs with high and low prolificacy. Wu et al. (2020) detected 20 candidate genes including PALM2-AKAP2, NANS, TRAF7, and PACSIN1 for body size through the runs of homozygosity (ROH) islands and integrated haplotype homozygosity score (iHS) methods in Diannan small-ear pigs. To date, although a total of 986 QTLs associated with reproductive traits have been detected (https://www.animalgenome.org/cgi-bin/QTLdb/SS/, last access: 28 October 2022), a limited number of genes were reported for each reproductive trait, which could explain only a small proportion of genetic variance (Manolio et al., 2009). Hence, more molecular markers and genes need to be further explored.
The aim of the present study was to detect potentially important genetic variants and genes associated with four litter traits in the Yorkshire pig population by performing a GWAS and selective sweep analysis.
2.1 Animals, phenotypic collection, and genetic parameter and estimated breeding value estimation
The pure-bred Yorkshire sows used in this study were raised under the same nutritional and feeding management at Fuyuefa Pig Breeding Farm (Kunming, China). Four litter traits – TNB, NBA, PBD, and LWB – were recorded for all sows. A total of 1969 litter records of 518 sows from parities 1 to 10 were collected during the year 2013 to 2020. Estimated breeding values (EBVs) for the four litter traits were calculated with the method of BLUP by tracing back four generations of pedigree information. The following animal repeatability model based on DMU software (http://dmiu.agrsci.dk, last access: 2 October 2022) was used to estimate the variance components and EBVs:
where Y was the vector of observation (including TNB, NBA, PBD, and LWB), fix was the vector of fixed effects (year–season–parity), add was the vector of additive genetic effects, sire was the random effects with breeding boars, per was the vector of individual permanent environmental effects, e was a vector of residuals, and X, Z, and P were incidence matrices associated with fix, add, and per, respectively. The farrowing parities were classified into four levels (parities 1, 2, 3, and more than 3) in order to reduce the imbalance among years and parities.
Based on the estimated variance components for each trait and their standard errors (SEs) by employing the average information restricted maximum likelihood (AI-REML) algorithm of DMU software, heritabilities (h2) and genetic correlations (rA) and their SEs were calculated. Among them, h2 and rA were calculated according to the formulas and , where , , , and were sequentially additive genetic variance, sire variance, permanent environmental variance, and residual variance of the same trait, and cov(a1,a2), and were the genetic covariance of two traits and genetic deviations of trait 1 and trait 2.
The EBVs of 518 sows for TNB were sorted from the lowest to the highest, and the highest and the lowest 15 individuals, namely high-prolificacy (HP) and low-prolificacy (LP) groups, were selected for subsequent selection signature analysis. The differences in the phenotypic values and EBVs between HP and LP groups were tested by the procedure GLM and TTEST in SAS9.2 (SAS Institute, Inc., Cary, North Carolina), respectively. The statistical model was described as follows:
For phenotypic values, significance tests were performed using model 2, where yijkl was the observed value of the trait, μ was the mean of the population, Parityj was an effect of parity, Yeark was an effect of the farrowing year, Seasonl was an effect of the farrowing season, and eijklm was an effect of residuals. For EBVs, a significance test was performed using model 3, where yij was the EBV of individual; μ and eij were the mean of EBV and residual effects, respectively; and Groupi was the grouping effect of HP and LP.
2.2 Genomic DNA extraction and genotyping
Totals of 518 individuals were genotyped and used for the GWAS analysis, of which 30 individuals were used for selection signature analysis. Genomic DNA was extracted from ear tissue following the manufacturer's instructions using the FinePure Universal Genomic DNA Kit. Then, DNA quality was detected using agarose gel electrophoresis and NanoDrop 2000 (Thermo Scientific, Waltham, MA, USA). Samples with clear and bright bands (no stray bands) and an A260 280 ratio between 1.7 and 2.1 were retained. Genotyping was performed using the Illumina Porcine SNP 50 BeadChip, which covered a comprehensive set of 51 315 single nucleotide polymorphisms (SNPs) distributed across the entire porcine genome. The genotyping was carried out by Beijing Compass Biotechnology Co., Ltd., located in Beijing, China.
2.3 Data quality control
For phenotypic data, descriptive statistics of the four litter traits were performed using the proc MEANS in SAS9.2 (SAS Institute, Inc., Cary, North Carolina) and tested for normality using the Shapiro.test function. For genotypic data, quality control was performed using the PLINK v1.9 software (Purcell et al., 2007). The filter criteria were as follows: (1) SNP call rate <0.90; (2) minor allele frequency <0.01; and (3) animals with a call rate <0.95; (4) for a Hardy–Weinberg equilibrium (HWE) test. Moreover, SNPs without positional information or those located on the sex chromosome were also removed from the dataset. Missing genotypes were imputed using Beagle5.2 (Browning et al., 2018).
2.4 Genome-wide association study
To control the false positive results caused by population stratification, gcta software was used to analyze the population structure. All quality-controlled SNPs were pruned using the indep-pairwise parameter in the PLINK v1.9 software (Purcell et al., 2007), and 10 201 independent SNPs were generated using a window size of 25 SNPs, a step size of 5 SNPs, and an r2 threshold of 0.4 (Gu et al., 2011). These 10 201 independent SNPs were then used for population stratification correction.
The GWAS analysis was implemented using a repeatability model on the basis of the GMAT software (Ning et al., 2019) for four traits. The statistical model was described as follows:
where Y was the phenotype vector (including TNB, NBA, PBD, and LWB); Xβ was the fixed effect, including the population structure effect of sow farrowing year, season, and parity; Zkγk was the marker effect to be tested; was the polygene effect; was the system environment effect; and was the residual effect. K in polygenic effects was the relatedness matrix inferred from markers. To avoid overcorrection, we used the previously calculated independent SNPs to determine the significance threshold; the threshold P value for genome-wide significant association was (0.05/10 201), and for suggestive association, it was (1/10 201) (Gu et al., 2011). Then, the genomic inflation factor λ was calculated using R.
2.5 Selective sweep analysis
To further illustrate the genetic differentiation between HP and LP sows, the following methods were performed. (1) Maximum likelihood (ML) trees were constructed using the MEGA7.0 software (Kumar et al., 2016) based on 41 314 genetic variants in each group and visualized using the ggtree package of R (Yu et al., 2018). (2) Principal component analysis (PCA) was performed using the PLINK v1.9 software (Purcell et al., 2007). The results were visualized using the ggplot2 package of R.
Subsequently, the selection signatures across genomes of HP and LP sows were detected using two statistics: the fixation index (FST) and the polymorphism level (θπ ratio). FST and θπ ratio were implemented using a 100 kb sliding window with a step size of 10 kb based on the vcftools software (Danecek et al., 2011). FST was the inbreeding coefficient of a subpopulation and was used for estimating the degree of pairwise genomic differentiation on candidate genes between pairs of subpopulations, and π was the expected heterozygosity per site derived from the average number of sequence differences in a group of samples (Lu et al., 2019). The top 5 % of regions were selected as candidate regions for selection by two methods. To make the results more reliable, the overlapping regions from the two methods were selected as key candidate regions.
2.6 Identification of candidate genes
For the GWAS, the potentially significant SNPs were annotated to the Sus Scrofa 10.2 reference genome assembly using the UCSC genome browser (Xu et al., 2019). Genes containing potentially significant SNPs or situated near the identified potentially significant SNPs (i.e., less than 300 kb away from potentially significant SNPs) were selected. For selective sweep analysis, genes located within the selective regions were regarded as candidate genes. Then, functional enrichment analysis of the gene ontology (GO) and Kyoto Encyclopedia of Genes and Genomes (KEGG) pathways was performed for the candidate genes by using the Database for Annotation, Visualization, and Integrated Discovery (DAVID) (Sherman et al., 2022).
3.1 Descriptive statistics of phenotypes
The results of a normality test showed that all the data of the four traits followed a normal distribution (P>0.88). The descriptive statistics of phenotypic values and EBV information for the four litter traits in this study are shown in Table 1. The phenotypic mean values of TNB, NBA, PBD, and LWB were 11.37, 9.67, 1.71, and 13.89, and the mean EBVs of the four traits were −0.09, −0.08, 0.01, and −0.68, respectively. There were large phenotypic variations in the four traits within the population. As shown in Table 2, the heritabilities of TNB, NBA, PBD, and LWB were 0.07, 0.06, 0.02, and 0.05, respectively. Strong positive genetic correlations were observed among the three traits, and the genetic correlation between TNB and NBA was 0.88.
Table 1Phenotypic values and EBVs of four litter traits.
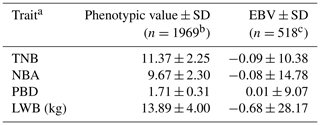
a TNB: total number born; NBA: number born alive; PBD: piglets born dead; LWB: litter weight born alive. b n: number of litters. c n: number of sows.
Table 2Trait heritabilities and genetic correlations among four litter traits.
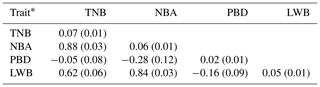
* The data on the diagonal are heritability (standard error), and data below the diagonal are genetic correlation (standard error) between two traits.
The phenotypic values and EBVs for the four litter traits of HP and LP groups were shown in Table 3. For TNB, the mean phenotypic value and EBV of the HP group were 14.85 and 19.91, which is significantly higher than for the LP group of 10.27 and −20.34 (P<0.001). The significance differences of NBA and LWB between the two groups were all consistent with those of TNB, but there were no significant differences (P>0.05) in PBD between HP and LP sows.
3.2 SNP identification
Through quality control, 296, 177, and 8890 SNPs were excluded from the raw genotype dataset due to missing genotype data, HWE test, and minor allele frequency (MAF), respectively (Fig. S1 in the Supplement). For selective sweep analysis, a total of 30 individuals and 41 314 SNPs remained for further analysis. For the GWAS analysis, 41 314 SNPs from 518 individuals were retained for subsequent analysis.
3.3 Genome-wide association study
A total of 14 SNPs that reached the suggestive significance level were detected to have an association with one of the tested litter traits (Table 4) and were defined as the potential SNPs for TNB, NBA, PBD, and LWB. And the λ values were 1.09, 1.09, 1.06, and 1.05 for the four traits, respectively. The results of population structure analysis were shown in Fig. S2. The manhattan plots and Q–Q plots across the whole genome for the four traits were shown in Figs. 1 and S3. By extending 300 kb downstream and upstream of the potential SNPs, a total of 49 different functional genes were discovered. Combining GO analysis, KEGG analysis, and the published literature, 13 functional genes were identified as promising candidates for litter traits (Table 4).
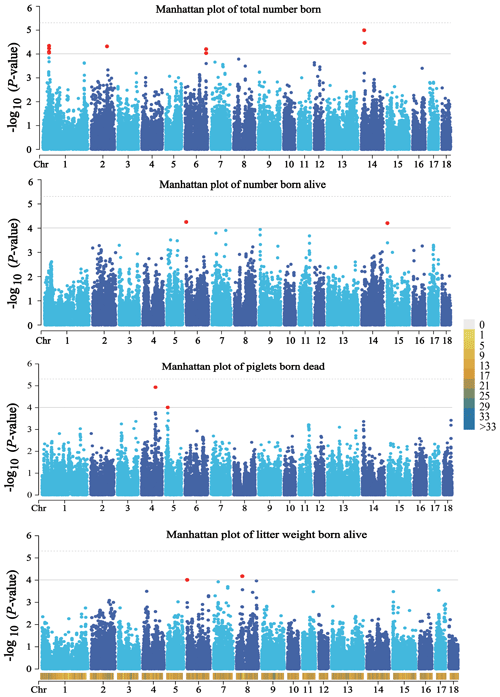
Figure 1Manhattan plots of the observed P values for four litter traits. The black horizontal lines indicate the suggestive significance () thresholds, and the horizontal dashed lines indicate the genome-wide significance () threshold. The red dots stand for the potential SNPs associated with the traits of TNB, NBA, PBD, and LWB. The different colors from top to bottom in the color scale stand for the number of SNPs on the chromosomes.
For TNB, we identified nine SNPs corresponding to eight related genes. The most significant SNP was rs80979178, located in the downstream region of the CLCN3 gene on chromosome 14. The other two SNPs (rs81393707 and rs81393705) were located in the introns of the CLIC1 and ZFYVE9 genes on chromosome 6. In addition, the remaining six SNPs were located on chromosomes 1, 2, 6, and 14, respectively. For NBA and LWB, a total of three SNPs corresponding to five genes were identified. The rs81478807 was located in the introns of the KIF5C gene on chromosome 15. Besides, GABRA2 and GABRA4 were found in the upstream region of rs81293800 located on chromosome 8. Interestingly, the rs81330557 located in the upstream region of the SPATA33 gene on chromosome 6 was significantly associated with NBA and LWB. For PBD, we identified two SNPs, which were located on chromosomes 4 and 5. Furthermore, GO and KEGG results showed that these genes were significantly enriched in some biological processes (BPs) and pathways related to solute transport, cell differentiation, embryonic development, and central regulation of reproduction. The functional genes of TNB were enriched in solute transport, and cell differentiation, such as CLCN3 and CLIC1, was enriched in chloride transport (GO:0006821) and chloride transmembrane transport (GO:1902476). Meanwhile, CLIC1 was enriched in the glutathione metabolic process (GO:0006749). Additionally, SCP2 and CBR4 were enriched in the fatty acid metabolism signal pathway (ssc01212). For LWB, the functional genes were involved in the central regulation process of reproduction; for example, GABRA2 and GABRA4 were enriched in the regulation of postsynaptic membrane potential (GO:0060078), synaptic transmission, and the GABAergic (GO:0051932) and gamma-aminobutyric acid signaling pathway (GO:0007214) (Table S1 in the Supplement).
3.4 Selective sweep analysis
Through phylogenetic tree analysis and PCA, significant genetic differentiation was detected between the HP and LP sows (Fig. 2). For the two groups, the PC1 and PC3 explained 13.83 % and 11.85 % of the total variations, respectively. Similar to PCA clustering analysis, ML trees also revealed clear population structures. Selective sweep analysis based on FST and θπ ratio showed many small selective regions. For θπ ratio, the average nucleotide diversity was and for HP and LP sows, respectively. And the 10 019 windows (top 5 %) were selected (Table S2). Meanwhile, the 10 157 selected regions were identified for FST (Table S3). Then, the overlapped regions corresponding to the two approaches (threshold, 5 %; FST, 0.15; π ratio, 2.13) were defined as the key selection signatures, which contained 256 genes (Table S4). Furthermore, functional enrichment analysis revealed that 32 genes were significantly enriched in seven GO BPs and one KEGG pathway (Table S5), including proteolysis (GO:0006508), the regulation of cell differentiation (GO:0045595), the positive regulation of phospholipid biosynthetic process (GO:0071073), and neuropeptide signaling pathway (GO:0007218) and so on. One KEGG pathway (FoxO signaling pathway) was identified as having an association with follicular development and ovulation. Combined with the published results, eight genes (NKAIN2, IGF1R, KISS1R, TYRO3, SPINT1, ADGRF5, APC2, and PTBP1) were identified as potential candidate genes (Fig. 3).
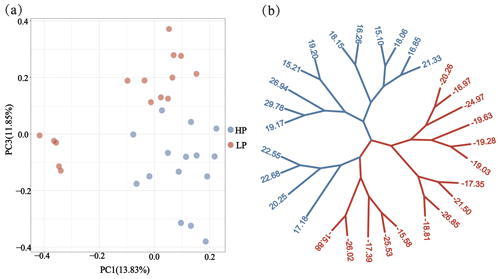
Figure 2Population structure and relationship between the HP and LP sows. (a) PCA clustering. (b) Maximum likelihood (ML) trees of the relationship between the HP and LP sows. Light blue and pink branches indicate sows in the HP and LP group, respectively, and the value of each leaf node represents the individual EBV of TNB.
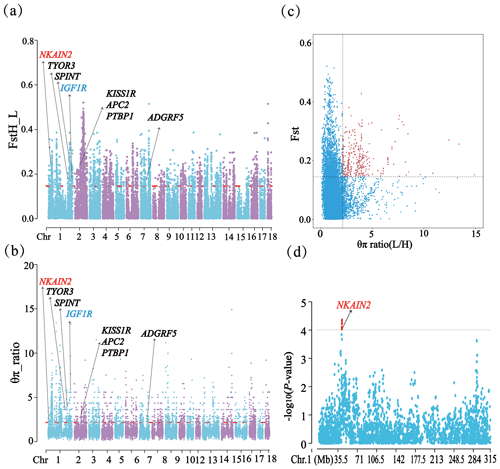
Figure 3FST, θπ ratio, and the GWAS for TNB on chromosome 1 related results in HP and LP sows. (a) Distribution of FST values among autosome chromosomes. (b) Distribution of θπ ratio values among autosome chromosomes. (c) The final selection regions based on two statistics. (d) Distribution of the GWAS for TNB on chromosome 1.
Litter traits such as TNB, NBA, PBD, and LWB are directly related to sow reproductive performance and affected pig production efficiency and economic profit. Therefore, it was extremely important to better understand the underlying genetic architecture of sow litter performance. In the present research, we used the Illumina Porcine SNP 50 BeadChip to genotype a Yorkshire pig population. Then, the GWAS based on the repeatability model and the selective sweep analysis were implemented.
For the GWAS, a total of 14 SNPs and 13 functional genes were significantly associated with litter traits, of which 12 genes were newly identified except for ZFYVE9.
The SNP rs81330557 on chromosome 6 was associated with NBA and LWB. This SNP was located within the upstream region of the spermatogenesis-associated 33 (SPATA33) gene, which is known as C16orf55 and is conserved among mammalian species. Chen et al. (2013) showed that SPATA33 was mainly expressed in spermatocytes, spermatogonia, and round spermatids and was related to spermatogenesis. Besides, it was reported that SPATA33 interacted with sperm calcine phosphatase through the PQIIIT sequence, and SPATA33 mutant mice displayed lower sperm motility due to the rigidity of the middle part of the sperm, impairing male fertility (Miyata et al., 2021).
The CLCN3 encodes a member of the voltage-gated chloride channel (ClC) family, which plays a role in the acidification and transmitter transmission of GABAergic synaptic vesicles. The GO and KEGG results showed that CLCN3 was significantly enriched in the BP of chloride transport. Besides, CLCN3 had previously been reported to be the most likely candidate gene involved in the human sperm volume regulation of the Cl− channel and promoted endometriosis cell migration and invasion (Yeung et al., 2005; Guan et al., 2016).
Two SNPs (rs81393707 and rs81393705) on chromosome 6 were also associated with TNB and were located within the intron of chloride intracellular channel 1 (CLIC1) and a FYVE-type zinc finger containing nine (ZFYVE9) genes, respectively. CLIC, a member of the p64 family, was mainly found in the nucleus and had chloride channel activity in the nuclear and plasma membranes. This gene was a sensor of cellular oxidation, involved in reactive oxygen species (ROS) production and was highly expressed in human embryos (Fagerberg et al., 2014; Goodchild et al., 2009; Averaimo et al., 2010). CLIC1 was enriched in two BP GO terms: chloride transport and glutathione metabolic process. Research has shown that the former might affect the stage-specific gradients of pH in the follicle cell, thereby regulating the intracellular environment, the development, and the function of follicles (Weiß and Bohrmann, 2019); the latter might be associated with pregnancy placental dysfunction, embryogenesis, and other processes (Beharier et al., 2021; Chen et al., 2022). There was report showed that CLIC1 expression accompanied by a low accumulation of ROS could promote embryonic development (Cajas et al., 2020).
ZFYVE9 also known as SARA was an FYVE-type zinc finger-containing protein (Di Guglielmo et al., 2003). Liu et al. (2013) showed that the knockout of ZFYVE9 affected the early embryonic development of zebrafish. In pigs, ZFYVE9 has been determined to be strongly associated with ovulation and the number of mummified foetuses by the GWAS (Onteru et al., 2012; Schneider et al., 2014). The results of association analysis by Li et al. (2020) also showed that ZFYVE9 was significantly correlated with TNB, and our result was consistent with these results.
In addition, an additional four candidate genes identified by the GWAS (HPF1, EPC2, GABRA2, and GABRA4) were also found to be linked to litter traits. HPF1 was a protein-coding gene that influenced chromatin binding activity and histone binding activity (Bilokapic et al., 2020). The decreased expression of HPF1 affected the early development of zebrafish (Zhang et al., 2018). EPC2 was mainly involved in the regulation of transcription by RNA polymerase II and has been reported to be associated with fertility in pigs (Chen et al., 2022). GABRA2 and GABRA4 are members of the GABA-A receptor gene family of heteromeric pentameric ligand-gated ion channels (Whiting et al., 1999) and significantly enriched in the BP of the regulation of postsynaptic membrane potential. Studies have shown that postsynaptic membrane potential regulation between neurons might affect the synthesis and release of reproductive hormones (DeFazio et al., 2019; Csillag et al., 2019). Therefore, the two genes might be related to reproductive hormone regulation and reproductive performance of pigs. It was speculated that the possible mechanism of the association of the two genes with reproductive performance regulated the secretion of estrogen by the regulation of brain development and transmission of neurotransmitters.
In the present study, only 14 potentially significant SNPs were detected by the GWAS. The main reason might be the limited size of the research population so that only SNPs or QTLs with large and moderate effects could be detected. Therefore, it is necessary to identify those SNPs or QTLs with small effects by expanding the sample size in future research.
Over the past 10 years, intensive breeding of the population has resulted in some genetic improvements in reproductive traits, especially in litter traits, which provided us with the opportunity to identify relevant candidate genes using selection signatures. According to earlier research, tests with extreme and moderate phenotypes in a single group could lessen the effects of the environment and the genetic differences among individuals (Jiang et al., 2016; Li et al., 2020). Therefore, to reveal the selective signatures of high prolificacy in Yorkshire pigs during domestication and breeding, we selected the region with the top 5 % FST and π ratio and identified 256 protein-coding genes. Thirty-two of these genes were significantly enriched in some BP GO terms; for example, KISS1R and TYRO3 were enriched in neuropeptide signaling pathway, SPINT1 was enriched in extracellular matrix organization, ADGRF5 was enriched in a positive regulation of phospholipid biosynthetic process, and APC2 and PTBP1 were enriched in the regulation of cell differentiation, which indicated that the functional genes were mainly related to the signal peptide transduction, embryonic development, and reproductive process. Additionally, five genes including IGF1R were significantly enriched in the FoxO signaling pathway, which was an important pathway affecting follicular development and ovulation (Lin et al., 2021).
Combining the selective sweep analysis and published results, eight genes (IGF1R, KISS1R, TYRO3, SPINT1, ADGRF5, APC2, PTBP1, and NKAIN2) were identified as candidate genes for litter traits. IGF1R was a receptor for an insulin-like growth factor, which was involved in the regulation of cell growth and survival control after activation. Experiments in mouse models have shown that IGF1R deficiency or low expression in the endometrium led to abnormal implantation of mouse embryos, resulting in poor fertility (Kang et al., 2015; Zhou et al., 2021). In pigs, through selective sweep analysis, IGF1R was identified as the key gene for high-prolificacy traits in Meishan pigs (Zhao et al., 2018). Zhang et al. (2022) also identified IGF1R as a candidate gene related to pig reproductive traits in a selective sweep analysis of Wannan black pigs and commercial pigs. The present result was consistent with the results reported. Given this background, IGF1R might be a key gene responsible for phenotypic differences in reproductive traits in pigs. CCND1 was a member of the highly conserved cyclin family. KISS1R was the receptor for the kisspeptin gene, and together they were involved in the neuroendocrine regulation of mammalian reproduction (Castano et al., 2009). A study also reported that KISS1R was able to promote oocyte maturation in vitro (Saadeldin et al., 2012). In pigs, it was reported that KISS1R might play a role in the physiological development of ovarian neovascularization (Basini et al., 2018). The TYRO3 gene was part of a three-member transmembrane receptor kinase receptor family that regulates spermatogenesis and male fertility in mice (Chen et al., 2009). In addition, TYRO3 modulated female reproduction by influencing gonadotropin-releasing hormone neuron survival and migration (Pierce et al., 2008). Recent studies have found that SPINT2 was a functionally relevant placental protease inhibitor and that low circulating SPINT1 was strongly associated with placental function defects (Kaitu'u-Lino et al., 2020; Murphy et al., 2021). It was inferred consequently that SPINT1 might influence sow litter size and piglet birth weight by regulating placental function. Polypyrimidine bundle-binding protein 1 (PTBP1) is a member of the heterogeneous nuclear ribonucleoproteins (hnRNPs) subfamily. It is an important cellular regulator of messenger RNAs and plays an important role in early embryonic development (Suckale et al., 2011). APC2 encodes a strongly conserved protein, which is preferentially expressed in post-mitotic neurons, and is involved in brain development through its regulation of neuronal migration and axon guidance (Almuriekhi et al., 2015). It was confirmed that APC2 plays a key role in regulating ovarian Wnt signaling pathways and ovarian homeostasis (Mohamed et al., 2019). ADGRF5, also known as GPR116, has been demonstrated to be associated with pig litter size through the GWAS (Sell-Kubiak et al., 2022). Our study further demonstrated that ADGRF5 is a potential candidate gene influencing litter traits in sows.
In addition, it is worth noting that the NKAIN2 gene was detected by three methods (GWAS, FST, and θπ ratio), indicating that it was strongly selected in the present population and might be significantly associated with litter traits. It could be attributed to its crucial functions in neurotransmitter transmission, energy metabolism, and ion transport (Blokland et al., 2022; Rudkowska et al., 2015). NKAIN2 encoding a transmembrane protein that interacts with the beta subunit of a sodium-/potassium-transporting ATPase may play essential roles in maintaining cell membrane potential, regulating neurotransmitter release, and ensuring proper ion homeostasis (Romania et al., 2013). These functions are vital for various reproductive processes in pigs. Neurotransmitters play a significant role in regulating reproductive functions, such as the modulation of the gonadotropin-releasing hormone (GnRH) and other hormones controlling the reproductive cycle. Previous studies have confirmed that germline alterations in the TCBA1 gene were associated with developmental delay and typical physiological features (Yue et al., 2006). Therefore, we speculated that NKAIN2's involvement in these processes might confer advantages in terms of fitness and reproductive success, leading to its strong selection in the population and potential association with litter traits. However, the function of this gene remains to be further explored.
In summary, a total of 20 promising candidate genes (NKAIN2, IGF1R, KISS1R, TYRO3, SPINT1, ADGRF5, APC2, PTBP1, CLCN3, CBR4, HPF1, FAM174A, SCP2, CLIC1, ZFYVE9, SPATA33, KIF5C, EPC2, GABRA2, and GABRA4) were identified as being associated with four litter traits (TNB, NBA, PBD, and LWB) in a Yorkshire pig population by using GWAS and selection signature methods. To the best of our knowledge, this study is the first to report that the NKAIN2 gene is associated with pig litter traits by integrating a GWAS and selective sweep analysis. These findings provide novel insights into the genetic basis of pig litter traits, which will be helpful for implementing marker-assisted selection and genomic selection to improve reproductive performances in the pig industry.
The datasets created and/or analyzed during the present study are not publicly accessible since the examined population is made up of the nucleus herd of the Yunnan Fuyuefa Livestock and Poultry Feeding Company Limited, but they are available from the corresponding author upon justifiable request.
The supplement related to this article is available online at: https://doi.org/10.5194/aab-66-357-2023-supplement.
SL and QC conceived and designed the experiments. ML, XW, YZ, and CJ determined the phenotypic data and collected the samples. YZ performed the experiment and processed and analyzed the data. ML, XW, and QC assisted with the processing of data. YZ and JL wrote the paper. QC and SL subsequently revised the paper. All authors have read and agreed to the published version of the paper.
The contact author has declared that none of the authors has any competing interests.
All animals used in the present study were handled and used by the standards for the care and use of laboratory animals established by the Ministry of Agriculture and Rural Affairs of China. All of the research was authorized by Yunnan Agricultural University's (YNAU, Kunming, China) ethical committee.
The research was approved by the ethics committee of Yunnan Agricultural University (YNAU, Kunming, China). Every effort was made to minimize suffering.
Publisher’s note: Copernicus Publications remains neutral with regard to jurisdictional claims made in the text, published maps, institutional affiliations, or any other geographical representation in this paper. While Copernicus Publications makes every effort to include appropriate place names, the final responsibility lies with the authors.
This research was funded by the Yunnan Swine Industry Technology System Program (grant no. 2019KJTX0013), Yunnan Province Science & Technology Specific Major Projects (grant nos. YNWR-CYJS-2018-056, 202102AE090039), the Yunnan Province Innovation Guidance and Technological Enterprise Cultivation Program (grant no. 202104BI090022), and the Yunnan Provincial Basic Research Program Project (grant no. 202001AU070113).
This paper was edited by Henry Reyer and reviewed by Liming Hou and two anonymous referees.
Almuriekhi, M., Shintani, T., Fahiminiya, S., Fujikawa, A., Kuboyama, K., Takeuchi, Y., Nawaz, Z., Nadaf, J., Kamel, H., Kitam, A. K., Samiha, Z., Mahmoud, L., Ben-Omran, T., Majewski, J., and Noda, M.: Loss-of-function mutation in APC2 causes sotos syndrome features, Cell Rep., 10, 1585–1598, https://doi.org/10.1016/j.celrep.2015.02.011, 2015.
Averaimo, S., Milton, R. H., Duchen, M. R., and Mazzanti, M.: Chloride intracellular channel 1 (CLIC1): Sensor and effector during oxidative stress, FEBS Lett., 584, 2076–2084, https://doi.org/10.1016/j.febslet.2010.02.073, 2010.
Basini, G., Grasselli, F., Bussolati, S., Ciccimarra, R., Maranesi, M., Bufalari, A., Parillo, F., and Zerani, M.: Presence and function of kisspeptin/KISS1R system in swine ovarian follicles, Theriogenology, 115, 1–8, https://doi.org/10.1016/j.theriogenology.2018.04.006, 2018.
Beharier, O., Kajiwara, K., and Sadovsky, Y.: Ferroptosis, trophoblast lipotoxic damage, and adverse pregnancy outcome, Placenta, 108, 32–38, https://doi.org/10.1016/j.placenta.2021.03.007, 2021.
Bilokapic, S., Suskiewicz, M. J., Ahel, I., and Halic, M.: Bridging of DNA breaks activates PARP2-HPF1 to modify chromatin, Nature, 585, 609–613, https://doi.org/10.1038/s41586-020-2725-7, 2020.
Blokland, G. A., Grove, J., Chen, C. Y., Cotsapas, C., Tobet, S., Handa, R., Ripke, S., Neale, B. M., Corvin, A., and Walters, J. T.: Sex-dependent shared and nonshared genetic architecture across mood and psychotic disorders, Biol. Psychiatry, 91, 102–117, https://doi.org/10.1016/j.biopsych.2021.02.972, 2022.
Bovo, S., Ballan, M., Schiavo, G., Ribani, A., Tinarelli, S., Utzeri, V. J., Dall'Olio, S., Gallo, M., and Fontanesi, L.: Single-marker and haplotype-based genome-wide association studies for the number of teats in two heavy pig breeds, Anim. Genet., 52, 440–450, https://doi.org/10.1111/age.13095, 2021.
Browning, B. L., Zhou, Y., and Browning, S. R.: A one-penny imputed genome from next-generation reference panels, Am. J. Hum. Genet., 103, 338–348, https://doi.org/10.1016/j.ajhg.2018.07.015, 2018.
Cajas, Y. N., Canon-Beltran, K., Ladron de Guevara, M., Millan de la Blanca, M. G., Ramos-Ibeas, P., Gutierrez-Adan, A., Rizos, D., and Gonzalez, E. M.: Antioxidant Nobiletin Enhances Oocyte Maturation and Subsequent Embryo Development and Quality, Int. J. Mol. Sci., 21, 5340, https://doi.org/10.3390/ijms21155340, 2020.
Castano, J. P., Martinez-Fuentes, A. J., Gutierrez-Pascual, E., Vaudry, H., Tena-Sempere, M., and Malagon, M. M.: Intracellular signaling pathways activated by kisspeptins through GPR54: do multiple signals underlie function diversity?, Peptides, 30, 10–15, https://doi.org/10.1016/j.peptides.2008.07.025, 2009.
Chen, H., Yi, M., Sheng, Y., Cheng, H., and Zhou, R.: A novel testis-enriched gene Spata33 is expressed during spermatogenesis, PLoS One, 8, e67882, https://doi.org/10.1371/journal.pone.0067882, 2013.
Chen, P. H., Tjong, W. Y., Yang, H. C., Liu, H. Y., Stern, A., and Chiu, D. T. Y.: Glucose-6-phosphate dehydrogenase, redox homeostasis and embryogenesis, Int. J. Mol. Sci., 23, 2017, https://doi.org/10.3390/ijms23042017, 2022.
Chen, Y., Wang, H., Qi, N., Wu, H., Xiong, W., Ma, J., Lu, Q., and Han, D.: Functions of TAM RTKs in regulating spermatogenesis and male fertility in mice, Reproduction, 138, 655–666, https://doi.org/10.1530/REP-09-0101, 2009.
Chen, Z., Ye, S., Teng, J., Diao, S., Yuan, X., Chen, Z., Zhang, H., Li, J., and Zhang, Z.: Genome-wide association studies for the number of animals born alive and dead in duroc pigs, Theriogenology, 139, 36–42, https://doi.org/10.1016/j.theriogenology.2019.07.013, 2019.
Chen, Z., Zhang, Z., Wang, Z., Zhang, Z., Wang, Q., and Pan, Y.: Heterozygosity and homozygosity regions affect reproductive success and the loss of reproduction: A case study with litter traits in pigs, Comput. Struct. Biotechnol. J., 20, 4060–4071, https://doi.org/10.1016/j.csbj.2022.07.039, 2022.
Csillag, V., Vastagh, C., Liposits, Z., and Farkas, I.: Secretin regulates excitatory GABAergic neurotransmission to GnRH neurons via retrograde NO signaling pathway in mice, Front. Cell. Neurosci., 13, 371, https://doi.org/10.3389/fncel.2019.00371, 2019.
D'Alessandro, E., Sottile, G., Sardina, M. T., Criscione, A., Bordonaro, S., Sutera, A. M., Zumbo, A., Portolano, B., and Mastrangelo, S.: Genome-wide analyses reveal the regions involved in the phenotypic diversity in Sicilian pigs, Anim. Genet., 51, 101–105, https://doi.org/10.1111/age.12887, 2020.
Danecek, P., Auton, A., Abecasis, G., Albers, C. A., Banks, E., DePristo, M. A., Handsaker, R. E., Lunter, G., Marth, G. T., Sherry, S. T., McVean, G., Durbin, R., and Genomes Project Analysis: The variant call format and VCFtools, Bioinformatics, 27, 2156–2158, https://doi.org/10.1093/bioinformatics/btr330, 2011.
DeFazio, R. A., Navarro, M. A., Adams, C. E., Milescu, L. S., and Moenter, S. M.: Estradiol enhances the depolarizing response to GABA and AMPA synaptic conductances in arcuate kisspeptin neurons by diminishing voltage-gated potassium currents, J. Neurosci., 39, 9532–9545, https://doi.org/10.1523/jneurosci.0378-19.2019, 2019.
Di Guglielmo, G. M., Le Roy, C., Goodfellow, A. F., and Wrana, J. L.: Distinct endocytic pathways regulate TGF-beta receptor signalling and turnover, Nat. Cell Biol., 5, 410–421, https://doi.org/10.1038/ncb975, 2003.
Fagerberg, L., Hallstrom, B. M., Oksvold, P., Kampf, C., Djureinovic, D., Odeberg, J., Habuka, M., Tahmasebpoor, S., Danielsson, A., Edlund, K., Asplund, A., Sjostedt, E., Lundberg, E., Szigyarto, C. A., Skogs, M., Takanen, J. O., Berling, H., Tegel, H., Mulder, J., Nilsson, P., Schwenk, J. M., Lindskog, C., Danielsson, F., Mardinoglu, A., Sivertsson, A., von Feilitzen, K., Forsberg, M., Zwahlen, M., Olsson, I., Navani, S., Huss, M., Nielsen, J., Ponten, F., and Uhlen, M.: Analysis of the human tissue-specific expression by genome-wide integration of transcriptomics and antibody-based proteomics, Mol. Cell. Proteomics, 13, 397–406, https://doi.org/10.1074/mcp.M113.035600, 2014.
Goodchild, S. C., Howell, M. W., Cordina, N. M., Littler, D. R., Breit, S. N., Curmi, P. M., and Brown, L. J.: Oxidation promotes insertion of the CLIC1 chloride intracellular channel into the membrane, Eur. Biophys. J., 39, 129–138, https://doi.org/10.1007/s00249-009-0450-0, 2009.
Gu, X., Feng, C., Ma, L., Song, C., Wang, Y., Da, Y., Li, H., Chen, K., Ye, S., Ge, C., Hu, X., and Li, N.: Genome-wide association study of body weight in chicken F2 resource population, PLoS One, 6, e21872, https://doi.org/10.1371/journal.pone.0021872, 2011.
Guan, Y. T., Huang, Y. Q., Wu, J. B., Deng, Z. Q., Wang, Y., Lai, Z. Y., Wang, H. B., Sun, X. X., Zhu, Y. L., Du, M. M., Zhu, L. Y., Chen, L. X., and Wang, L. W.: Overexpression of chloride channel-3 is associated with the increased migration and invasion ability of ectopic endometrial cells from patients with endometriosis, Hum. Reprod., 31, 986–998, https://doi.org/10.1093/humrep/dew034, 2016.
He, L. C., Li, P. H., Ma, X., Sui, S. P., Gao, S., Kim, S. W., Gu, Y. Q., Huang, Y., Ding, N. S., and Huang, R. H.: Identification of new single nucleotide polymorphisms affecting total number born and candidate genes related to ovulation rate in Chinese Erhualian pigs, Anim. Genet., 48, 48–54, https://doi.org/10.1111/age.12492, 2017.
Hidalgo, A. M., Lopes, M. S., Harlizius, B., and Bastiaansen, J. W.: Genome-wide association study reveals regions associated with gestation length in two pig populations, Anim. Genet., 47, 223–226, https://doi.org/10.1111/age.12395, 2016.
Holm, B., Bakken, M., Klemetsdal, G., and Vangen, O.: Genetic correlations between reproduction and production traits in swine, J. Anim. Sci., 82, 3458–3464, https://doi.org/10.2527/2004.82123458x, 2004.
Jiang, J., Gao, Y., Hou, Y., Li, W., Zhang, S., Zhang, Q., and Sun, D.: Whole-genome resequencing of holstein bulls for indel discovery and identification of genes associated with milk composition traits in dairy cattle, PLoS One, 11, e0168946, https://doi.org/10.1371/journal.pone.0168946, 2016.
Jiang, Y., Tang, S., Xiao, W., Yun, P., and Ding, X.: A genome-wide association study of reproduction traits in four pig populations with different genetic backgrounds, Asian Austral. J. Anim., 33, 1400–1410, https://doi.org/10.5713/ajas.19.0411, 2020.
Kaitu'u-Lino, T. J., MacDonald, T. M., Cannon, P., Nguyen, T. V., Hiscock, R. J., Haan, N., Myers, J. E., Hastie, R., Dane, K. M., Middleton, A. L., Bittar, I., Sferruzzi-Perri, A. N., Pritchard, N., Harper, A., Hannan, N. J., Kyritsis, V., Crinis, N., Hui, L., Walker, S. P., and Tong, S.: Circulating SPINT1 is a biomarker of pregnancies with poor placental function and fetal growth restriction, Nat. Commun., 11, 2411, https://doi.org/10.1038/s41467-020-16346-x, 2020.
Kang, Y. J., Lees, M., Matthews, L. C., Kimber, S. J., Forbes, K., and Aplin, J. D.: MiR-145 suppresses embryo-epithelial juxtacrine communication at implantation by modulating maternal IGF1R, J. Cell Sci., 128, 804–814, https://doi.org/10.1242/jcs.164004, 2015.
Kumar, S., Stecher, G., and Tamura, K.: MEGA7: Molecular evolutionary genetics analysis version 7.0 for bigger datasets, Mol. Biol. Evol., 33, 1870–1874, https://doi.org/10.1093/molbev/msw054, 2016.
Li, X., Ye, J., Han, X., Qiao, R., Li, X., Lv, G., and Wang, K.: Whole-genome sequencing identifies potential candidate genes for reproductive traits in pigs, Genomics, 112, 199–206, https://doi.org/10.1016/j.ygeno.2019.01.014, 2020.
Lin, J., Guan, L., Ge, L., Liu, G., Bai, Y., and Liu, X.: Nanopore-based full-length transcriptome sequencing of Muscovy duck (Cairina moschata) ovary, Poultry Sci., 100, 101246, https://doi.org/10.1016/j.psj.2021.101246, 2021.
Liu, N., Li, Z., Pei, D., and Shu, X.: Zfyve9a regulates the proliferation of hepatic cells during zebrafish embryogenesis, Int. J. Dev. Biol., 57, 773–778, https://doi.org/10.1387/ijdb.130065xs, 2013.
Lu, K., Wei, L., Li, X., Wang, Y., Wu, J., Liu, M., Zhang, C., Chen, Z., Xiao, Z., Jian, H., Cheng, F., Zhang, K., Du, H., Cheng, X., Qu, C., Qian, W., Liu, L., Wang, R., Zou, Q., Ying, J., Xu, X., Mei, J., Liang, Y., Chai, Y. R., Tang, Z., Wan, H., Ni, Y., He, Y., Lin, N., Fan, Y., Sun, W., Li, N. N., Zhou, G., Zheng, H., Wang, X., Paterson, A. H., and Li, J.: Whole-genome resequencing reveals Brassica napus origin and genetic loci involved in its improvement, Nat. Commun., 10, 1154, https://doi.org/10.1038/s41467-019-09134-9, 2019.
Manolio, T. A., Collins, F. S., Cox, N. J., Goldstein, D. B., Hindorff, L. A., Hunter, D. J., McCarthy, M. I., Ramos, E. M., Cardon, L. R., Chakravarti, A., Cho, J. H., Guttmacher, A. E., Kong, A., Kruglyak, L., Mardis, E., Rotimi, C. N., Slatkin, M., Valle, D., Whittemore, A. S., Boehnke, M., Clark, A. G., Eichler, E. E., Gibson, G., Haines, J. L., Mackay, T. F., McCarroll, S. A., and Visscher, P. M.: Finding the missing heritability of complex diseases, Nature, 461, 747–753, https://doi.org/10.1038/nature08494, 2009.
Miyata, H., Oura, S., Morohoshi, A., Shimada, K., Mashiko, D., Oyama, Y., Kaneda, Y., Matsumura, T., Abbasi, F., and Ikawa, M.: SPATA33 localizes calcineurin to the mitochondria and regulates sperm motility in mice, P. Natl. Acad. Sci. USA., 118, e2106673118, https://doi.org/10.1073/pnas.2106673118, 2021.
Mohamed, N. E., Hay, T., Reed, K. R., Smalley, M. J., and Clarke, A. R.: APC2 is critical for ovarian WNT signalling control, fertility and tumour suppression, BMC Cancer, 19, 677, https://doi.org/10.1186/s12885-019-5867-y, 2019.
Moscatelli, G., Dall'Olio, S., Bovo, S., Schiavo, G., Kazemi, H., Ribani, A., Zambonelli, P., Tinarelli, S., Gallo, M., Bertolini, F., and Fontanesi, L.: Genome-wide association studies for the number of teats and teat asymmetry patterns in Large White pigs, Anim. Genet., 51, 595–600, https://doi.org/10.1111/age.12947, 2020.
Murphy, C. N., Walker, S. P., MacDonald, T. M., Keenan, E., Hannan, N. J., Wlodek, M. E., Myers, J., Briffa, J. F., Romano, T., Roddy Mitchell, A., Whigham, C. A., Cannon, P., Nguyen, T. V., Kandel, M., Pritchard, N., Tong, S., and Kaitu'u-Lino, T. J.: Elevated circulating and placental SPINT2 is associated with placental dysfunction, Int. J. Mol. Sci., 22, 7467, https://doi.org/10.3390/ijms22147467, 2021.
Ning, C., Wang, D., Zhou, L., Wei, J., Liu, Y., Kang, H., Zhang, S., Zhou, X., Xu, S., and Liu, J. F.: Efficient multivariate analysis algorithms for longitudinal genome-wide association studies, Bioinformatics, 35, 4879–4885, https://doi.org/10.1093/bioinformatics/btz304, 2019.
Onteru, S. K., Fan, B., Du, Z. Q., Garrick, D. J., Stalder, K. J., and Rothschild, M. F.: A whole-genome association study for pig reproductive traits, Anim. Genet., 43, 18–26, https://doi.org/10.1111/j.1365-2052.2011.02213.x, 2012.
Pierce, A., Bliesner, B., Xu, M., Nielsen-Preiss, S., Lemke, G., Tobet, S., and Wierman, M. E.: Axl and Tyro3 modulate female reproduction by influencing gonadotropin-releasing hormone neuron survival and migration, Mol. Endocrinol., 22, 2481–2495, https://doi.org/10.1210/me.2008-0169, 2008.
Purcell, S., Neale, B., Todd-Brown, K., Thomas, L., Ferreira, M. A., Bender, D., Maller, J., Sklar, P., de Bakker, P. I., Daly, M. J., and Sham, P. C.: PLINK: a tool set for whole-genome association and population-based linkage analyses, Am. J. Hum. Genet., 81, 559–575, https://doi.org/10.1086/519795, 2007.
Romania, P., Castellano, A., Surace, C., Citti, A., De Ioris, M. A., Sirleto, P., De Mariano, M., Longo, L., Boldrini, R., Angioni, A., Locatelli, F., and Fruci, D.: High-resolution array CGH profiling identifies Na/K transporting ATPase interacting 2 (NKAIN2) as a predisposing candidate gene in neuroblastoma, PLoS One, 8, e78481, https://doi.org/10.1371/journal.pone.0078481, 2013.
Rudkowska, I., Pérusse, L., Bellis, C., Blangero, J., Després, J. P., Bouchard, C., and Vohl, M. C.: Interaction between common genetic variants and total fat intake on low-density lipoprotein peak particle diameter: a genome-wide association study, J. Nutrigenet. Nutrige., 8, 44–53, https://doi.org/10.1159/000431151, 2015.
Saadeldin, I. M., Koo, O. J., Kang, J. T., Kwon, D. K., Park, S. J., Kim, S. J., Moon, J. H., Oh, H. J., Jang, G., and Lee, B. C.: Paradoxical effects of kisspeptin: it enhances oocyte in vitro maturation but has an adverse impact on hatched blastocysts during in vitro culture, Reprod. Fert. Develop., 24, 656–668, https://doi.org/10.1071/RD11118, 2012.
Schneider, J. F., Nonneman, D. J., Wiedmann, R. T., Vallet, J. L., and Rohrer, G. A.: Genomewide association and identification of candidate genes for ovulation rate in swine, J. Anim. Sci., 92, 3792–3803, https://doi.org/10.2527/jas.2014-7788, 2014.
See, G. M., Trenhaile-Grannemann, M. D., Spangler, M. L., Ciobanu, D. C., and Mote, B. E.: A genome-wide association study for gestation length in swine, Anim. Genet., 50, 539–542, https://doi.org/10.1111/age.12822, 2019.
Sell-Kubiak, E., Knol, E. F., and Lopes, M.: Evaluation of the phenotypic and genomic background of variability based on litter size of Large White pigs, Genet. Sel. Evol., 54, 1, https://doi.org/10.1186/s12711-021-00692-5, 2022.
Sherman, B. T., Hao, M., Qiu, J., Jiao, X., Baseler, M. W., Lane, H. C., Imamichi, T., and Chang, W.: DAVID: a web server for functional enrichment analysis and functional annotation of gene lists (2021 update), Nucleic Acids Res., 50, W216–W221, https://doi.org/10.1093/nar/gkac194, 2022.
Suckale, J., Wendling, O., Masjkur, J., Jager, M., Munster, C., Anastassiadis, K., Stewart, A. F., and Solimena, M.: PTBP1 is required for embryonic development before gastrulation, PLoS One, 6, e16992, https://doi.org/10.1371/journal.pone.0016992, 2011.
Uzzaman, M. R., Park, J. E., Lee, K. T., Cho, E. S., Choi, B. H., and Kim, T. H.: A genome-wide association study of reproductive traits in a Yorkshire pig population, Livestock Science, 209, 67–72, https://doi.org/10.1016/j.livsci.2018.01.005, 2018.
Weiß, I. and Bohrmann, J.: Electrochemical patterns during Drosophila oogenesis: ion-transport mechanisms generate stage-specific gradients of pH and membrane potential in the follicle-cell epithelium, BMC Dev. Biol., 19, 12, https://doi.org/10.1186/s12861-019-0192-x, 2019.
Wernersson, R., Schierup, M. H., Jorgensen, F. G., Gorodkin, J., Panitz, F., Staerfeldt, H. H., Christensen, O. F., Mailund, T., Hornshoj, H., Klein, A., Wang, J., Liu, B., Hu, S., Dong, W., Li, W., Wong, G. K., Yu, J., Wang, J., Bendixen, C., Fredholm, M., Brunak, S., Yang, H., and Bolund, L.: Pigs in sequence space: a 0.66X coverage pig genome survey based on shotgun sequencing, BMC Genomics, 6, 70, https://doi.org/10.1186/1471-2164-6-70, 2005.
Whiting, P. J., Bonnert, T. P., McKernan, R. M., Farrar, S., Le Bourdelles, B., Heavens, R. P., Smith, D. W., Hewson, L., Rigby, M. R., Sirinathsinghji, D. J., Thompson, S. A., and Wafford, K. A.: Molecular and functional diversity of the expanding GABA-A receptor gene family, Ann. N.Y. Acad. Sci., 868, 645–653, https://doi.org/10.1111/j.1749-6632.1999.tb11341.x, 1999.
Wu, F., Sun, H., Lu, S., Gou, X., Yan, D., Xu, Z., Zhang, Z., Qadri, Q. R., Zhang, Z., Wang, Z., Chen, Q., Li, M., Wang, X., Dong, X., Wang, Q., and Pan, Y.: Genetic diversity and selection signatures within Diannan Small-ear pigs revealed by next-generation sequencing, Front. Genet., 11, 733, https://doi.org/10.3389/fgene.2020.00733, 2020.
Wu, P., Yang, Q., Wang, K., Zhou, J., Ma, J., Tang, Q., Jin, L., Xiao, W., Jiang, A., Jiang, Y., Zhu, L., Li, X., and Tang, G.: Single step genome-wide association studies based on genotyping by sequence data reveals novel loci for the litter traits of domestic pigs, Genomics, 110, 171–179, https://doi.org/10.1016/j.ygeno.2017.09.009, 2018.
Xu, L., Yang, L., Zhu, B., Zhang, W., Wang, Z., Chen, Y., Zhang, L., Gao, X., Gao, H., Liu, G. E., and Li, J.: Genome-wide scan reveals genetic divergence and diverse adaptive selection in Chinese local cattle, BMC Genomics, 20, 494, https://doi.org/10.1186/s12864-019-5822-y, 2019.
Yeung, C. H., Barfield, J. P., and Cooper, T. G.: Chloride channels in physiological volume regulation of human spermatozoa, Biol. Reprod., 73, 1057–1063, https://doi.org/10.1095/biolreprod.105.044123, 2005.
Yu, G., Lam, T. T., Zhu, H., and Guan, Y.: Two Methods for mapping and visualizing associated data on phylogeny using Ggtree, Mol. Biol. Evol., 35, 3041–3043, https://doi.org/10.1093/molbev/msy194, 2018.
Yue, Y., Stout, K., Grossmann, B., Zechner, U., Brinckmann, A., White, C., Pilz, D. T., and Haaf, T.: Disruption of TCBA1 associated with a de novo t(1;6)(q32.2;q22.3) presenting in a child with developmental delay and recurrent infections, J. Med. Genet., 43, 143–147, https://doi.org/10.1136/jmg.2004.029660, 2006.
Zhang, W., Li, X., Jiang, Y., Zhou, M., Liu, L., Su, S., Xu, C., Li, X., and Wang, C.: Genetic architecture and selection of Anhui autochthonous pig population revealed by whole genome resequencing, Front. Genet., 13, 1022261, https://doi.org/10.3389/fgene.2022.1022261, 2022.
Zhang, Z., Chen, Z., Ye, S., He, Y., Huang, S., Yuan, X., Chen, Z., Zhang, H., and Li, J.: Genome-wide association study for reproductive traits in a Duroc pig population, Animals, 9, 732, https://doi.org/10.3390/ani9100732, 2019.
Zhang, Z., Sun, H., Chen, Y., Cao, T., Songyang, Z., Huang, J., and Huang, Y.: Analysis of hpf1 expression and function in early embryonic development of zebrafish, Dev. Genes Evol., 228, 141–147, https://doi.org/10.1007/s00427-018-0608-9, 2018.
Zhao, P., Yu, Y., Feng, W., Du, H., Yu, J., Kang, H., Zheng, X., Wang, Z., Liu, G. E., Ernst, C. W., Ran, X., Wang, J., and Liu, J. F.: Evidence of evolutionary history and selective sweeps in the genome of Meishan pig reveals its genetic and phenotypic characterization, Gigascience, 7, giy058, https://doi.org/10.1093/gigascience/giy058, 2018.
Zhou, C., Lv, M., Wang, P., Guo, C., Ni, Z., Bao, H., Tang, Y., Cai, H., Lu, J., Deng, W., Yang, X., Xia, G., Wang, H., Wang, C., and Kong, S.: Sequential activation of uterine epithelial IGF1R by stromal IGF1 and embryonic IGF2 directs normal uterine preparation for embryo implantation, J. Mol. Cell Biol., 13, 646–661, https://doi.org/10.1093/jmcb/mjab034, 2021.