the Creative Commons Attribution 4.0 License.
the Creative Commons Attribution 4.0 License.
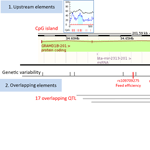
The microRNA gene bta-mir-2313 in cattle: an atlas of regulatory elements and an association analysis with growth and carcass traits in the Slovenian Simental cattle breed
Špela Malovrh
Tanja Kunej
Milena Kovač
Peter Dovč
MicroRNAs (miRNA) are a class of non-coding RNAs important in posttranscriptional regulation of target genes. Regulation requires complementarity between the target mRNA and the miRNA region responsible for their recognition and binding, also called the seed region. Previous studies have proven that expression profiles and genetic variations of miRNA genes (miR-SNP; SNP – single nucleotide polymorphism) and their target sites (miR-TS-SNPs) have an impact on phenotypic variation and disease susceptibility in human, animal models, and livestock. MicroRNA-associated polymorphisms therefore represent biomarker potential for phenotypic traits in livestock. Effects of miRNA gene polymorphisms on phenotypic traits have been studied in several animal species but much less in cattle. The aim of the present study was therefore to analyze the genetic variability in the bta-mir-2313 gene and test associations with growth and carcass traits of the Slovenian Simmental cattle breed. Additionally, validated and predicted genomic information related to the miRNA gene bta-mir-2313 has been obtained and presented as an atlas of miRNA regulatory elements. Sanger sequencing has been used for biomarker development and genotyping of 145 animals of Slovenian dual-purpose Simmental cattle. Out of nine known polymorphisms located within pre-miRNA regions, one mature miRNA seed SNP was polymorphic in the Slovenian Simmental cattle breed. An additional three polymorphisms were identified within the flanking pri-miRNA regions. There was no significant effect of polymorphisms on 18 tested fattening and carcass traits; however, validated polymorphisms could now be tested in association with other traits in other cattle populations. The microRNA gene bta-mir-2313 warrants further genetic and functional analyses since it overlaps with a large number of quantitative trait loci (QTL), has over 3100 predicted targets and highly polymorphic mature seed regions, and is located within protein-coding gene GRAMD1B, previously associated with production traits in cattle. Mature miRNA seed SNPs present important genomic loci for functional studies because they could affect the gain/loss of downstream targets and should be systematically studied in cattle.
- Article
(900 KB) - Full-text XML
-
Supplement
(276 KB) - BibTeX
- EndNote
MicroRNAs (miRNAs) are non-coding RNAs (ncRNAs), about 21 nucleotides in length, which bind to target mRNAs and regulate gene expression (Bartel, 2004). By binding to different target gene regions, including 3′-untranslated region (3′-UTR), 5′-UTR, promoter, or coding sequences, they repress or activate translation (reviewed in Kunej et al., 2012). During miRNA biogenesis, precursors undergo two cleavage steps performed by Drosha, cleaving of primary miRNA (pri-miRNA) to produce precursor miRNA (pre-miRNA), and Dicer, cleaving pre-miRNA to create mature miRNA (Bartel, 2004; Lee et al., 2002). The key binding location for miRNA target recognition is the seed region, positioned two to seven or eight nucleotides from the 5′-end of the miRNA (Lewis et al., 2005; Piletič and Kunej, 2017). About half of miRNA genes reside within protein-coding host genes, and if in the same orientation, they share their transcriptional mechanisms (Godnic et al., 2013).
MicroRNA dysregulation has been reported to be associated with several traits in cattle, for example resistance to mastitis in dairy cattle (Li et al., 2017). Additionally, various miRNA regulome polymorphisms – within miRNA genes (miR-SNPs; single nucleotide polymorphisms), regulatory regions (miR-rSNPs), target sites (miR-TS-SNPs), and silencing machinery (miR-SM-SNPs) have also been associated with phenotypic traits and diseases (Obsteter et al., 2015; Georges et al., 2007; Hrovatin and Kunej, 2018). It is estimated that each miRNA regulates 200 target genes (Krek et al., 2005); therefore, it is expected that miR-SNPs have a more profound effect than those within miRNA target sites (Sun et al., 2009). Our previous in silico genome-wide screening of the genetic variability in miRNA genes in 15 animal species revealed that based on the current database releases, miRNA genes are most polymorphic in cattle (Zorc et al., 2015). Additionally, cattle miRNA genes also comprise several consecutive polymorphisms, referred to also as double and multiple nucleotide polymorphisms (DNPs and MNPs) (Jevsinek Skok et al., 2013). Mature miRNA seed SNPs could also cause an alteration of the seed region and cause the formation of a novel seed region belonging to a different miRNA gene (Jevsinek Skok et al., 2013). However, the genetic variability in miRNA genes in cattle has not been systematically experimentally analyzed, and several polymorphisms have unknown validation status. For the analysis, we selected bta-mir-2313 since this miRNA was shown to be one of the most polymorphic miRNA genes in cattle (Zorc et al., 2015) and it has also been proposed as one of the causes for regulatory mechanisms of mastitis (Li et al., 2015). For bta-mir-2313 we therefore obtained relevant genomic information from various databases and performed experimental validation of polymorphisms and genotyping and an association analysis in the Slovenian Simental cattle breed.
2.1 Databases and bioinformatics tools
Relevant genomic information related to bta-mir-2313 has been obtained and presented as an atlas of regulatory elements as described previously (Zorc and Kunej, 2015). Genomic location and genetic variability related to the bta-mir-2313 gene were extracted from the Ensembl genomic browser, release 91 (Zerbino et al., 2018), and miRBase, release 21 (Kozomara and Griffiths-Jones, 2011). The validation status of miRNA polymorphisms was extracted from dbSNP, Ensembl, and PubMed databases. The overlap of miRNA genomic locations with locations of SNPs from the commercial DNA microarrays “Bovine HD 770K” Illumina, USA, was analyzed using SNPchiMp v.3 (Nicolazzi et al., 2015). Overlapping quantitative trait loci (QTL) were downloaded from QTLdb, release 34 (December 2017) (Hu et al., 2016). Criteria for the CpG (cytosine–phosphate–guanine) island search were used as set by default parameters in MethPrimer tool: island size > 100; GC (guanine, cytosine) percent > 50.0; observed/expected > 0.6. (Li and Dahiya, 2002). Experimentally validated miRNA–target interactions were screened in TarBase v.7.0 (Paraskevopoulou et al., 2016), MirTarBase 6.0 (Chou et al., 2016), and miRecords (Xiao et al., 2009). The mature miRNA seed region and predicted targets were extracted from TargetScan 7.0 (Agarwal et al., 2015). The identification of mature miRNA seed polymorphisms causing the formation of novel seed region belonging to different miRNA gene was performed using the TargetScan 7.0 tool as described previously (Jevsinek Skok et al., 2013).
2.2 Cattle samples and experimental validation of bta-mi-2313 polymorphisms
Cattle samples (n= 145) were obtained within the frame of the national progeny test for Slovenian dual-purpose Simmental cattle in which all bulls were slaughtered at the end. DNA from the semen of eight sires was extracted using the DNeasy Blood & Tissue DNA extraction kit (Qiagen, Düseldorf, Germany). Polymerase chain reaction (PCR) primers were selected using the Primer 3 tool (http://frodo.wi.mit.edu/, last access: 4 November 2011) (Untergasser et al., 2012): bta-mir-2313-F – 5′-GCACAGACTCTCAGCCACTG-3′; bta-mir-2313-R – 5′-CTGACTGAGGCTCTCGCTCT-3′ comprising pre-miRNA and flanking regions of the miRNA gene. Conditions for the PCR were 94 ∘C for 10 min, 30 cycles of 94 ∘C for 1 min, 63 ∘C for 1 min, and 72 ∘C for 1 min, followed by a further 10 min extension at 72 ∘C. The PCR products were submitted for sequencing to the Macrogen Europe Sequencing service (Amsterdam, The Netherlands).
2.3 Statistical analysis
Association analysis was performed between four polymorphisms of the miRNA gene bta-mir-2313 and haplotypes, as effects and the 18 fattening and carcass quality traits in bulls from the progeny test station were tested. Phenotypes and polymorphisms were known for 145 bulls. Included traits were live weight at slaughter, carcass weight, live daily gain, net daily gain, dressing percentage, conformation index, weights of front and hind quarters, percentage of front and hind quarters on the right side of the carcass, weight of lean meat, daily gain of lean meat, fat weight, bones weight, percentage of valuable cuts, lean meat percentage, lean meat percentage in valuable cuts, and the ratio between lean meat and fat. Bulls were weighed before slaughter. At slaughter, the carcass was weighed and chest depth and carcass length were measured. Daily gains were calculated, as well as dressing percentage and conformation index: live daily gain = (weight at slaughter − birth weight) / age at slaughter; net daily gain = (carcass weight − birth weight) / age at slaughter; dressing percentage = carcass weight / weight at slaughter × 100; conformation index = carcass weight / (chest depth × carcass length) × 1000. In dissection, first the right side of bull carcass is cut into quarters between the sixth and seventh ribs and then cut to the following cuts: chuck, shoulder, front shank, rib roast, back, loin, tenderloin, brisket, rib, flank, leg, and hind shank. The cuts were dissected into separable muscle tissue (lean meat), separable subcutaneous fat and intermuscular fat tissue (fat), separable tendon, and separable bone. Later, weights, and percentage of tissues were calculated, as well as the ratios between tissues. Statistical analyses were performed by the ordinary least square method as implemented in the GLM procedure in SAS/STAT (SAS Institute Inc., 2013). It was planned to use the Bonferroni multiple comparison correction because of an increased risk of a type I error when making multiple statistical tests simultaneously. The critical p value was set at 0.0028 due to the Bonferroni correction; usually, a given critical p value (0.05) was divided by 18 (number of traits).
Two-step analysis was performed to study the miRNA gene bta-mir-2313 in cattle. In the first step all known validated and predicted miRNA-associated genomic information was retrieved, integrated, and presented as an atlas of miRNA regulatory elements, consisting of miRNA gene upstream, downstream, and overlapping elements. In the second step, we analyzed the genetic variability in the miRNA gene bta-mir-2313 in association with carcass traits in cattle. The nucleotide sequence flanking the miRNA gene was sequenced and four polymorphisms were identified and tested for association with 18 production traits in Slovenian Simmental cattle.
3.1 Obtaining genomic information from genomic databases
We first obtained relevant genomic information related to bta-mir-2313 (Gene ID: 100313327) from various genomic databases and bioinformatics tools. The data were presented as an atlas of miRNA gene regulatory elements, consisting of miRNA gene upstream (polymorphisms, CpG islands), overlapping (host gene, polymorphisms, and QTL), and downstream genomic elements (miRNA targets). The collected data include validated and predicted genomic information (Table 1). The miRNA gene bta-mir-2313 is located within the intron 2 of the GRAMD1B (GRAM (glucosyltransferases, Rab-like GTPase activators and myotubularin) domain containing 1B) gene at the same orientation relative to its host gene (Fig. 1). The pre-miRNA region of bta-mir-2313 comprises 74 bp and includes nine polymorphisms deposited in the genomic browsers (Fig. 2a). Those nine pre-miRNA polymorphisms include four SNPs, two consecutive polymorphisms forming DNP, and three consecutive polymorphisms forming MNP. The miRNA gene bta-mir-2313 consists of two seed regions, both having two polymorphisms: one DNP in the 5′ mature miRNA seed region and two SNPs in the 3′ mature miRNA seed region. Out of four seed SNPs present in the databases, three have an unknown validation status, and polymorphism rs41761413 has been validated by multiple, independent submissions to the refSNP cluster. A comparison of known polymorphisms with the SNPchiMp database revealed that commercial DNA-microarray SNPs currently do not overlap with the bta-mir-2313 gene.
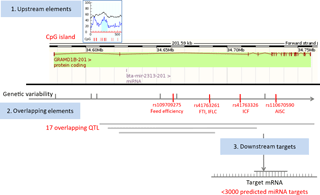
Figure 1Map of miRNA bta-mir-2313 regulatory elements. The miRNA gene bta-mir-2313 is located within the intron 2 of the GRAMD1B gene at the same orientation relative to its host gene. Four functional polymorphisms are located downstream of the miRNA gene, three within intron 2 and one within intron 7 of the GRAMD1B gene. The miRNA gene has over 3000 predicted target genes. Legend: FTI – fertility index; IFLC – days from first to last insemination in cows; ICF – length in days of the interval from calving to first insemination; AISC – number of inseminations per conception in cows.
To analyze genomic overlaps between the miRNA gene and QTL, we downloaded data from the QTLdb database using the genomic location of the miRNA gene bta-mir-2313 as a query: 15:34628901-34628974. Seventeen QTL matched the query (Supplement Table S1). QTL overlapping the miRNA gene span large regions, the most distant breakpoints spanning from Chr15:20 bp (QTL ID: 19626) to Chr15: 63 827 753 bp (QTL ID: 20794), and therefore, it is not possible to draw conclusions on their association with the miRNA gene. QTL are associated with diverse phenotypic traits, including body weight, height, teat placement, marbling score, and tridecylic acid content. Peaks of overlapping QTL range from 41.14 to 84.89. The QTL that is the closest to the miRNA gene bta-mir-2313 is mapped between 34 177 504 and 35 177 124 bp and is associated with the fertility index (QTL ID: 62361).
To identify mature miRNA seed polymorphisms causing the formation of a novel seed regions corresponding to a different miRNA gene, we screened all available mature miRNA seed regions deposited in the TargetScan database. The miRNA gene bta-mir-2313 consists of two seed regions, both having two polymorphisms. We checked all possible combinations of nucleotides present in the seed regions and found one polymorphism, which causes the formation of the seed region belonging to a different miRNA gene. Polymorphism rs41761413C > T is located within the bta-miR-2313-3p seed region CAGUUCC, and it causes the formation of a novel seed region CAGUUCU which corresponds to a miRNA gene bta-miR-3600 (Supplement Fig. S1). The number of predicted targets differs between the two seed regions. bta-miR-2313-3p has 3393 target transcripts, and bta-miR-3600 has 3789 predicted transcripts.
To identify upstream miRNA gene regulators we performed a CpG island search. There was one CpG island present around 4900 bp upstream of the miRNA gene and comprising 104 bp. To identify miRNA gene downstream genomic elements, we collected information related to validated and predicted miRNA targets. According to three databases collecting experimentally validated miRNA–target interactions (miRTarBase, TarBase, and miRecords), currently experimentally validated interactions for bta-miR-2313 are not yet known. For example, the current version of the miRTarBase lists 242 miRNA–target interactions between 21 miRNAs and 111 target genes in cattle; however, the current version of the database does not include data for the gene bta-miR-2313. On the other hand, according to the TargetScan tool, bta-miR-2313 has a large number (> 3100) of predicted targets (Table 1).
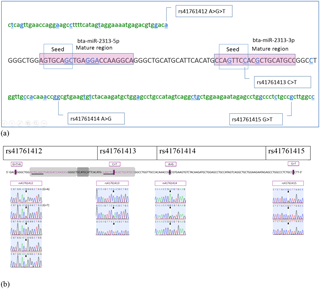
Figure 2Genomic location and polymorphisms of the bta-mir-2313 gene in cattle. (a) bta-mir-2313 pre-miRNA sequence and flanking pri-miRNA sequence with polymorphisms extracted from the Ensembl database. The four polymorphisms validated in our tested population are marked in squares. (b) Experimental validation of polymorphisms rs41761413 located within the mature seed region and three additional SNPs within the pri-miRNA region (rs41761412, rs41761414, and rs41761415).
3.2 Marker development and genotyping of polymorphisms in Slovenian Simmental cattle
Out of nine pre-miRNA polymorphisms deposited in genomic databases, rs41761413 has been polymorphic in Slovenian dual-purpose Simmental cattle. This polymorphism is located within the mature miRNA seed region. Additionally, three polymorphisms were identified within the flanking gene region (pri-miRNA): one upstream (rs41761412) and two downstream of the miRNA gene (rs41761414 and rs41761415) (Fig. 2b). These three pri-miRNA polymorphisms have previously been deposited in genomic browsers; however, their validation status is unknown and allele frequency has not yet been determined. These four polymorphisms were genotyped in 145 animals. Frequencies of genotypes for four polymorphisms tested in 145 animals are shown in Table 2.
3.3 Association analysis
The four polymorphisms identified were tested for association with 18 traits. All four polymorphisms were in Hardy–Weinberg equilibrium (the p values from the chi-square test were 0.77, 0.84, 0.80, and 0.97). We can assume that natural or artificial selection does not favor one phenotype over another and that migrations and mutations have no effect. There was no significant effect of polymorphisms on growth and carcass traits in our data, even if 0.05 is taken as critical p value. However, there were some trends (p values between 0.07 and 0.12) for the effect of rs41761414 and rs41761415 on live daily gain, net daily gain, and daily gain of lean meat and for the effect of rs41761414 on the ratio between lean meat and fat. The polymorphisms explained around 4 % of phenotypic variation for those traits.
The miRNA bta-mir-2313 has been reported as one of the potential biomarkers for the diagnosis of mastitis in dairy cows. This miRNA has been found to be differentially expressed in bovine mammary gland tissue samples from the Staphylococcus aureus-induced mastitis group and controls (Li et al., 2015). Our study did not reveal associations between bta-mir-2313 polymorphisms and 18 phenotypic traits tested. The miRNA gene bta-mir-2313 is located within the intron 2 of the protein-coding host gene GRAMD1B. However, the miRNA host gene GRAMD1B has previously been associated with production and reproduction traits in cattle. This gene has been associated with the feed efficiency (Serão et al., 2013), female fertility index (FTI), days from first to last insemination in cows (IFLC), length in days of the interval from calving to first insemination (ICF), and the number of inseminations per conception in cows (AISC) (Höglund et al., 2015).
Several genes involved in growth and muscle development have been shown to be under the control of miRNAs (Jin et al., 2016). Polymorphisms within miRNA targets in cattle were found to be associated with an altered phenotype (Gao et al., 2014); however, polymorphisms within miRNA genes have not yet been systematically investigated. While the mature miRNA seed region is the most important region for miRNA–miRNA target hybridization and accurate miRNA function, these genomic regions present important functional candidates. Therefore, polymorphisms within the mature miRNA seed region could affect miRNA target recognition and also create new targets. Additionally, miRNA polymorphisms could also cause the formation of a seed region, belonging to a different miRNA and therefore the formation of a novel miRNA gene, as we reported previously for the example of the cattle miRNA gene bta-mir-29b-2/bta-mir-29e (Jevsinek Skok et al., 2013). The present study revealed another example of mature miRNA seed SNP causing the formation of a novel seed region: the substitution C > T caused the formation of a bta-miR-2313 > bta-miR-3600 and also an increase in the number of predicted miRNA targets. These predicted miRNA targets need to be experimentally validated to further explore an effect of the polymorphism on miRNA function.
Beside SNPs, the bta-miR-2313 gene also includes one DNP and one MNP, composed of two and three consecutive polymorphisms, respectively. Multiple nucleotide polymorphisms include more than one nucleotide and therefore have a greater chance of effect on miRNA function. If DNP or MNPs are present within mature miRNA seed regions, mature miRNA regions are no longer entirely complementary to their targets. Consequently, these polymorphisms could completely change the miRNA seed region, enabling the hybridization of this particular miRNA with a completely new set of targets. However, several miRNA polymorphisms have not yet been validated and do not have known minor allele frequency (MAF); therefore, they could be sequencing errors or mutations present in only a few individuals. There is no information available regarding the MAF of bta-miR-2313 seed polymorphisms; therefore, this region should be sequenced.
Our previous genome-wide in silico screening revealed that among the known 808 miRNA genes in cattle, only 12 had polymorphisms included in commercial SNP arrays, including only two miRNAs with mature miRNA seed SNPs (Zorc et al., 2015). The aim of the present study is also to raise awareness amongst researchers that miRNA polymorphisms should be included in SNP chips, especially if located within mature miRNA seed regions or other genomic elements including host genes and QTL. For example, our previous study revealed some important genomic hotspots in the cattle genome, including an overlap between three different types of genes (miRNA, snoRNA, and a protein-coding gene): bta-mir-1839, SCARNA15, and MROH1 (Zorc et al., 2015).
For a more efficient design of functional studies and biomarker development, a complete regulatory network comprising all miRNA interactions should be considered. Previously, we developed an atlas of the regulatory network for human miRNA genes hsa-miR-34 (Strmsek and Kunej, 2014) and hsa-miR-1306 (Zorc and Kunej, 2015). Additionally, we also proposed the classification of miRNA-related sequence variants (Hrovatin and Kunej, 2018), including miRNA upstream regulation (Piletič and Kunej, 2016) and downstream targets (Piletič and Kunej, 2017). However, the present study revealed that there are less databases and bioinformatics tools available for analysis of cattle miRNA genes. The tools are also species-specific and therefore cannot be used for analyses in all species. For example, several tools are available for the prediction of transcription factor binding sites in humans but not in cattle. An increasing amount of genomic data is being produced, and with more information from future publications and databases, it will be possible to develop a more complete map of miRNA regulatory elements and more efficient biomarker prioritization for experimental validation.
In conclusion, the current study did not reveal any associations of miRNA polymorphisms with phenotypic traits; however, validated miRNA-associated polymorphisms could now be tested in association with other traits in other cattle populations. The miRNA gene bta-mir-2313 warrants further genetic and functional analyses since it overlaps with a large number of QTL, has over 3100 predicted downstream targets, and has highly polymorphic mature seed regions, including one polymorphism causing the formation of a novel miRNA gene affecting the number of putative miRNA targets. The miRNA gene is located within the protein-coding gene GRAMD1B, previously associated with production traits in cattle. Mature miRNA seed SNPs present important genomic loci for functional studies because they could affect the gain/loss of downstream targets. The results of the present study will be useful for further functional studies investigating the influence of miRNA polymorphisms on miRNA function, in particular on target recognition. Biomarkers based on miRNA polymorphisms contribute to the study of phenotypic properties of interest in animal husbandry and medicine.
Data associated with this study are found in the Supplement of this paper.
The supplement related to this article is available online at: https://doi.org/10.5194/aab-61-271-2018-supplement.
TK and SM performed analyses, interpreted the data, and wrote the draft of the paper, and MK and PD supervised the study and revised the final version of the paper.
The authors declare that they have no conflict of interest.
This work was supported by the Slovenian Research Agency projects J4-9252,
P4-0220, J4-4306, and V4-1084 and the Joint Core Breeding Program (STRP).
Edited by: Steffen Maak
Reviewed by: Runjun Yang and one anonymous referee
Agarwal, V., Bell, G. W., Nam, J. W., and Bartel, D. P.: Predicting effective microRNA target sites in mammalian mRNAs, Elife, 4, e05005, https://doi.org/10.7554/eLife.05005, 2015.
Bartel, D. P.: MicroRNAs: genomics, biogenesis, mechanism, and function, Cell, 116, 281–297, 2004.
Chou, C. H., Chang, N. W., Shrestha, S., Hsu, S. D., Lin, Y. L., Lee, W. H., Yang, C. D., Hong, H. C., Wei, T. Y., Tu, S. J., Tsai, T. R., Ho, S. Y., Jian, T. Y., Wu, H. Y., Chen, P. R., Lin, N. C., Huang, H. T., Yang, T. L., Pai, C. Y., Tai, C. S., Chen, W. L., Huang, C. Y., Liu, C. C., Weng, S. L., Liao, K. W., Hsu, W. L., and Huang, H. D.: miRTarBase 2016: updates to the experimentally validated miRNA-target interactions database, Nucleic Acids Res., 44, D239–D247, https://doi.org/10.1093/nar/gkv1258, 2016.
Gao, Q., Ju, Z., Zhang, Y., Huang, J., Zhang, X., Qi, C., Li, J., Zhong, J., Li, G., and Wang, C.: Association of TNP2 gene polymorphisms of the bta-miR-154 target site with the semen quality traits of Chinese Holstein bulls, PLoS One, 9, e84355, https://doi.org/10.1371/journal.pone.0084355, 2014.
Georges, M., Coppieters, W., and Charlier, C.: Polymorphic miRNA-mediated gene regulation: contribution to phenotypic variation and disease, Curr. Opin. Genet. Dev., 17, 166–176, https://doi.org/10.1016/j.gde.2007.04.005, 2007.
Godnic, I., Zorc, M., Jevsinek Skok, D., Calin, G. A., Horvat, S., Dovc, P., Kovac, M., and Kunej, T.: Genome-wide and species-wide in silico screening for intragenic MicroRNAs in human, mouse and chicken, PLoS One, 8, e65165, https://doi.org/10.1371/journal.pone.0065165, 2013.
Hrovatin, K. and Kunej, T.: Classification of miRNA-related sequence variations, Epigenomics, 10, 463–481, https://doi.org/10.2217/epi-2017-0126, 2018.
Höglund, J. K., Buitenhuis, B., Guldbrandtsen, B., Lund, M. S., and Sahana, G.: Genome-wide association study for female fertility in Nordic Red cattle, BMC Genet., 16, 110, https://doi.org/10.1186/s12863-015-0269-x, 2015.
Hu, Z. L., Park, C. A., and Reecy, J. M.: Developmental progress and current status of the Animal QTLdb, Nucleic Acids Res., 44, D827–D833, https://doi.org/10.1093/nar/gkv1233, 2016.
Jevsinek Skok, D., Godnic, I., Zorc, M., Horvat, S., Dovc, P., Kovac, M., and Kunej, T.: Genome-wide in silico screening for microRNA genetic variability in livestock species, Anim. Genet., 44, 669–677, https://doi.org/10.1111/age.12072, 2013.
Jin, Y., Cai, H., Liu, J., Lin, F., Qi, X., Bai, Y., Lei, C., Chen, H., and Lan, X.: The 10 bp duplication insertion/deletion in the promoter region within paired box 7 gene is associated with growth traits in cattle, Arch. Anim. Breed., 59, 469–476, https://doi.org/10.5194/aab-59-469-2016, 2016.
Kozomara, A. and Griffiths-Jones, S.: miRBase: integrating microRNA annotation and deep-sequencing data, Nucleic Acids Res., 39, D152–D157, https://doi.org/10.1093/nar/gkq1027, 2011.
Krek, A., Grün, D., Poy, M. N., Wolf, R., Rosenberg, L., Epstein, E. J., MacMenamin, P., da Piedade, I., Gunsalus, K. C., Stoffel, M., and Rajewsky, N.: Combinatorial microRNA target predictions, Nat. Genet., 37, 495–500, https://doi.org/10.1038/ng1536, 2005.
Kunej, T., Godnic, I., Horvat, S., Zorc, M., and Calin, G. A.: Cross talk between microRNA and coding cancer genes, Cancer J., 18, 223–231, https://doi.org/10.1097/PPO.0b013e318258b771, 2012.
Lee, Y., Jeon, K., Lee, J. T., Kim, S., and Kim, V. N.: MicroRNA maturation: stepwise processing and subcellular localization, EMBO J., 21, 4663–4670, 2002.
Lewis, B. P., Burge, C. B., and Bartel, D. P.: Conserved seed pairing, often flanked by adenosines, indicates that thousands of human genes are microRNA targets, Cell, 120, 15–20, https://doi.org/10.1016/j.cell.2004.12.035, 2005.
Li, L. C. and Dahiya, R.: MethPrimer: designing primers for methylation PCRs, Bioinformatics, 18, 1427–1431, 2002.
Li, R., Zhang, C. L., Liao, X. X., Chen, D., Wang, W. Q., Zhu, Y. H., Geng, X. H., Ji, D. J., Mao, Y. J., Gong, Y. C., and Yang, Z. P.: Transcriptome microRNA profiling of bovine mammary glands infected with Staphylococcus aureus, Int. J. Mol. Sci., 16, 4997–5013, https://doi.org/10.3390/ijms16034997, 2015.
Li, Z., Wang, H., Chen, L., Zhai, M., Chen, S., Li, N., and Liu, X.: Identification and expression analysis of miR-144-5p and miR-130b-5p in dairy cattle, Arch. Anim. Breed., 60, 199–204, https://doi.org/10.5194/aab-60-199-2017, 2017.
Nicolazzi, E. L., Caprera, A., Nazzicari, N., Cozzi, P., Strozzi, F., Lawley, C., Pirani, A., Soans, C., Brew, F., Jorjani, H., Evans, G., Simpson, B., Tosser-Klopp, G., Brauning, R., Williams, J. L., and Stella, A.: SNPchiMp v.3: integrating and standardizing single nucleotide polymorphism data for livestock species, BMC Genomics, 16, 283, https://doi.org/10.1186/s12864-015-1497-1, 2015.
Obsteter, J., Dovc, P., and Kunej, T.: Genetic variability of microRNA regulome in human, Mol. Genet. Genomic. Med., 3, 30–39, https://doi.org/10.1002/mgg3.110, 2015.
Paraskevopoulou, M. D., Vlachos, I. S., and Hatzigeorgiou, A. G.: DIANA-TarBase and DIANA Suite Tools: Studying Experimentally Supported microRNA Targets, Curr. Protoc. Bioinformatics, 55, 12.14.11–12.14.18, https://doi.org/10.1002/cpbi.12, 2016.
Piletič, K. and Kunej, T.: MicroRNA epigenetic signatures in human disease, Arch. Toxicol., 90, 2405–2419, https://doi.org/10.1007/s00204-016-1815-7, 2016.
Piletič, K. and Kunej, T.: Minimal Standards for Reporting microRNA:Target Interactions, OMICS, 21, 197–206, https://doi.org/10.1089/omi.2017.0023, 2017.
SAS Institute Inc.: Version 9.4 of the SAS System for Unix and Linux, Cary, NC, SAS, Institute Inc., 2013.
Serão, N. V., González-Peña, D., Beever, J. E., Faulkner, D. B., Southey, B. R., and Rodriguez-Zas, S. L.: Single nucleotide polymorphisms and haplotypes associated with feed efficiency in beef cattle, BMC Genet., 14, 94, https://doi.org/10.1186/1471-2156-14-94, 2013.
Strmsek, Z. and Kunej, T.: Data integration of 104 studies related with microRNA epigenetics revealed that miR-34 gene family is silenced by DNA methylation in the highest number of cancer types, Discoveries, 2, e18, https://doi.org/10.15190/d.2014.10, 2014.
Sun, G., Yan, J., Noltner, K., Feng, J., Li, H., Sarkis, D. A., Sommer, S. S., and Rossi, J. J.: SNPs in human miRNA genes affect biogenesis and function, RNA, 15, 1640–1651, https://doi.org/10.1261/rna.1560209, 2009.
Untergasser, A., Cutcutache, I., Koressaar, T., Ye, J., Faircloth, B. C., Remm, M., and Rozen, S. G.: Primer3–new capabilities and interfaces, Nucleic Acids Res., 40, e115, https://doi.org/10.1093/nar/gks596, 2012.
Xiao, F., Zuo, Z., Cai, G., Kang, S., Gao, X., and Li, T.: miRecords: an integrated resource for microRNA-target interactions, Nucleic Acids Res., 37, D105–D110, https://doi.org/10.1093/nar/gkn851, 2009.
Zerbino, D. R., Achuthan, P., Akanni, W., Amode, M. R., Barrell, D., Bhai, J., Billis, K., Cummins, C., Gall, A., Girón, C. G., Gil, L., Gordon, L., Haggerty, L., Haskell, E., Hourlier, T., Izuogu, O. G., Janacek, S. H., Juettemann, T., To, J. K., Laird, M. R., Lavidas, I., Liu, Z., Loveland, J. E., Maurel, T., McLaren, W., Moore, B., Mudge, J., Murphy, D. N., Newman, V., Nuhn, M., Ogeh, D., Ong, C. K., Parker, A., Patricio, M., Riat, H. S., Schuilenburg, H., Sheppard, D., Sparrow, H., Taylor, K., Thormann, A., Vullo, A., Walts, B., Zadissa, A., Frankish, A., Hunt, S. E., Kostadima, M., Langridge, N., Martin, F. J., Muffato, M., Perry, E., Ruffier, M., Staines, D. M., Trevanion, S. J., Aken, B. L., Cunningham, F., Yates, A., and Flicek, P.: Ensembl 2018, Nucleic Acids Res., 46, D754–D761, https://doi.org/10.1093/nar/gkx1098, 2018.
Zorc, M. and Kunej, T.: Development of Integrative Map of MicroRNA Gene Regulatory Elements, Microrna, 2015.
Zorc, M., Obsteter, J., Dovc, P., and Kunej, T.: Genetic variability of microRNA genes in 15 animal species, Journal of Genomics, 3, 51–56, https://doi.org/10.7150/jgen.11246, 2015.